I’ve spent the better part of the past year thinking about the application and commercialization of Artificial Intelligence (AI), especially within customer experience and contact center domains. The pace of innovation and the rate at which capabilities have expanded has been absolutely mind-blowing – and it’s been fun and challenging to keep up. But, as radical and as rapid as the change has been, my take is that it will follow a very familiar pattern on how it gets actually incorporated into real business use cases on a wide scale.
I’m heavily influenced on this by Clayton Christensen’s theories, notably his model of disruptive innovation and his modularity theory and by Ben Thompson’s Stratechery (which in turn has a pretty strong Christensen bias, though he notably argues convincingly that this doesn’t apply to consumer products). We are navigating through the first of three evolutionary stages in AI solutions, which can be thought of as methods, monoliths, and modules. If you understand where we are in the process, you can make better choices about how to allocate resources to leverage the power of generative AI in each phase.
Methods – You are Here!
The advent of any groundbreaking technology typically ushers in a bespoke phase characterized by custom-built and custom-implemented solutions. In the realm of AI, this phase is dominated by what can be collectively referred to as “methods” – solutions born from domain expertise and deliberate design patterns. This might be best illustrated by what it isn’t – today’s generative AI is not a product. Sounds funny from a guy whose title is Chief Product Officer and is charged with bringing AI enabled and enabling products to market. But that is where we are today, i.e. despite some compelling marketing campaigns to the contrary, you can’t buy a generative AI product and just drop it in and expect it to work (today, anyway). Keep in mind, many firms will try to sell you exactly that today. It just isn’t going to work without real effort, expertise, and iteration. But, you can start building and be successful. You just need to focus on the methods. The patterns and the people who understand them.
A powerful example of this custom build deployment is Klarna‘s AI rollout. Collaborating directly with OpenAI, Klarna embarked on an 18-month journey of designing, building, and refining their AI capabilities, a process that culminated in a successful implementation without the procurement of an off-the-shelf product. This approach, which prioritizes tailored solutions over generalized offerings, is particularly appealing to firms aiming to leverage AI as a disruptive innovation, often resulting in cost-effective, down-market strategies. It took time and effort, but they are already realizing the benefit. If your firm can make that kind of internal pivot, that’s the best way to approach and achieve in the first phase.
If your firm doesn’t have the internal talent or organizational capacity to conceive of, create, and deploy (and then iterate, and iterate, and iterate), then you’ll need a trusted partner to bring the patterns, practices, and people to you. Convenient side note – that’s what we do at Waterfield Tech. But if you want to capitalize on the capabilities today, be prepared to do the work. And if you follow the space, I think you’ll see the real success stories at this point the game are bespoke and driven be firm-specific needs.
Monoliths – Coming soon!
The subsequent phase in the evolution of AI solutions is marked by the emergence of integrated platforms that offer tightly coupled, comprehensive solutions. This phase, which I refer to as “monoliths,” is currently (rightly) being pursued by Contact Center as a Service (CCaaS) platforms, enterprise software firms, and Customer Relationship Management (CRM) systems. These monolithic solutions, designed for ease of deployment within existing platforms, sacrifice flexibility to cater to a broad audience. However, they can effectively serve niche or vertical markets, provided these markets are large enough to justify the development of a dedicated solution.
We already see this happening in some other domains. A great example is in the enterprise offices suite, e.g, Microsoft‘s suite-wide integration of Copilot throughout Office, Teams, and Dynamics. It has both the pros (it’s already built in and easy to use – why open another window for ChatGPT when summary and knowledgebase info are in the window you’re working in) and the cons (it solves some problems well and others not at all).
It’s worth noting that for most of the firms that pursue this course, it will be an effort to capture the change unleashed by generative AI from running wild as a truly disruptive innovation, and corral it into a sustaining innovation. You can see this most clearly in the way these guys attempt to preserve the business model and extract incremental value, as opposed to flipping the business model. Whether or not it works will depend on how well they execute on building the monolith and if the incremental value is enough to prevent customers from switching.
Modules – Endgame
The final and most mature phase of AI solution evolution is characterized by modularity. This phase emerges when the industry has developed sufficient standards and API normalization to allow for the assembly of diverse solution components through simple configuration rather than complex customization. The modular phase represents the likely endgame for AI solution evolution, where flexibility, efficiency, and customization converge to meet the diverse needs of users and industries. It seems like we are pretty far away from this segment, but once the integration points are mature we should expect to see competition drive down price with good enough results. And at the rate things are changing, perhaps we aren’t that far away after all.
What I suspect will be different about the modular phase in this disruptive cycle is that the modules will appear primarily as options within the big cloud computing platforms, i.e. AWS, Azure, and GCP will continue to be platforms but also serve as aggregators for various options for each piece of the stack for GAI solutions (but that is a whole different article).
The trajectory of AI application and commercialization, from bespoke methods through integrated monoliths to modular solutions, reflects a broader pattern of technological evolution observed across various industries. As we navigate these stages, the key to success lies in understanding the inherent trade-offs and opportunities each phase presents. By anticipating the shift towards modularity, firms can strategically position themselves to harness the full potential of AI, fostering innovation that aligns with evolving market demands and technological capabilities.
About the Author
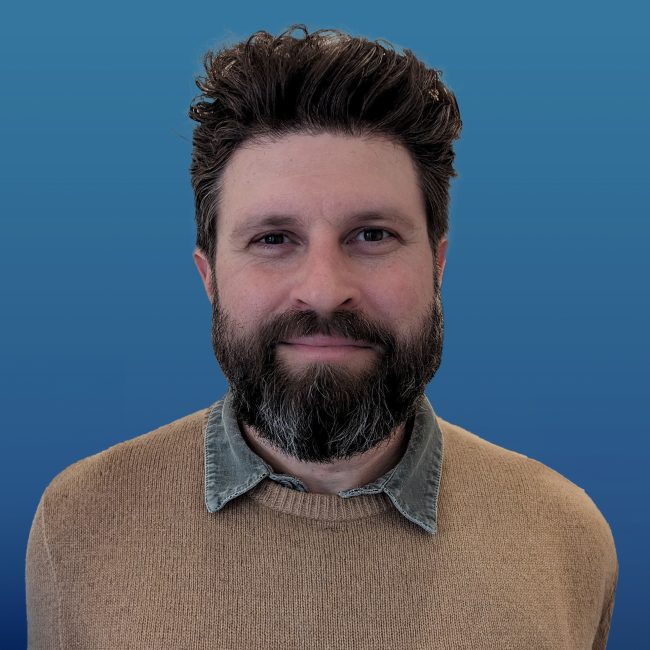
Michael Fisher
Chief Product Officer
Waterfield Tech
Michael Fisher (a.k.a Fish) has built and led product, technology, and operations teams in organizations ranging from early-stage startups to publicly traded companies. Over the past twenty years, he has guided companies from inception to sale and through mergers, acquisitions, and complex integrations.