Alexa, Black Friday, and boiled tea(?) – A chat with an expert.
I had the opportunity to sit down with Sarah Reitsma, Director of UI at Waterfield Technologies, to discuss conversational AI in the contact center and how it is helping industries of all sizes and types provide better user experiences, streamline processes, and meet the expectations of today’s customer.
Ruth:
Thank you for joining me today to talk about conversational AI, Sarah! To start, is there anything in particular that you’ve been seeing lately regarding conversational AI that is surprising to you?
Sarah
I think one of the things is that people are throwing around the terminology, but they don’t really know what it means. So, it sounds basic, but really, what is it? What’s the whole point of it? Why would someone want it?
This technology works well in cases where only a conversation will do, and you can’t easily add numeric values for things that you want to enter over the phone.
For instance, let’s say that you want to use natural language to capture something open-ended. Certain things, in a numeric world, work well. If I need somebody to make a payment, I can easily ask them for their payment amount, and they can enter that on the phone.
But let’s say that you are a municipality, and you are taking calls from people who are complaining about things like broken stoplights, graffiti, and a splash pad that’s broken. Think about things the city maintains. The set is much bigger than a finite number of options. That’s where conversational AI technologies are working really well.
“Today, however, you don’t have to choose from my limited list of options. With natural language, you can simply tell me what you want.”
Ruth
That’s great! There’s nothing better than talking about real-life examples… It makes it easy for organizations to relate to a specific pain point such as how to assist callers in resolving issues when a simple “press one for this” or “press two for that” doesn’t work. I can see how challenging it could be to try to guess every reason why a person may be calling or how they’ll phrase their request when you’re moving through system design. Many companies find themselves restricted by the number of options they can offer the caller.
Sarah
Right! Take an industry like financial. They want to allow people to interact with them, not having to choose an option from a menu, but just to say something like “I need to reorder checks,” or “I need to transfer money from my checking to my savings account.” Those little pieces of what people say, there’s so much context in them versus just pressing a number.
We started these systems with pressing a number. “Press one if you want to pay your bill, press two if you wanted to do this, press three, etc.” And then we went to “Which of the following do you want do you want to do? Pay a bill, transfer funds, or check your account balance?” Even with that, you still have a limited set of options, but you can speak what you want. Today, however, you don’t have to choose from my limited list of options. With natural language, you can simply tell me what you want.
Ruth
I’ve gotten used to speaking requests over the phone in everyday life.
Sarah
Yes, people are easily adapting to using these systems because of the virtual assistants such as Amazon Alexa or Google Home—most people have something like that sitting in their house. And so, they’ve learned how to interact with these things now. We can take those same concepts and apply them over the phone.
People are learning that they can say a lot more than just the single types of commands that have only been supported in the past. And they’re trying to interact with our systems like that. You might call in and say, “I want to transfer money from my checking to my savings account.” Or you may say “I want to transfer $500 from my checking to my savings account.” You’ve just provided a bunch of information that can be used to finish your transaction. So that is really automating the things that were too hard to automate before because we couldn’t capture what the person wanted to do with just numbers. It also streamlines the process dramatically because you can get to whatever task you’re trying to complete much faster.
So if you say, “I want to transfer money from my checking to my savings,” it’s going to look at your account and see that maybe you only have one checking account and one savings account, eliminating the need to ask you about those accounts. Obviously, it will need to verify your identity and make sure that you have permission to transfer money around and that sort of thing. But once it is past that point, depending on the level of information you said at the beginning, it doesn’t need to go through that whole interaction. So based on the level of information that a certain customer gives, you may have a really different interaction with the system than someone that just says “transfer funds” at the beginning. This technology can meet you where you are and not have to re-ask a bunch of questions, because you already provided a lot of context coming in. That’s a huge part of conversational AI—it can look at that whole snippet of text that they provided you and pull out all the important things without discarding any of the context. It can keep all of that and use it later in the call.
Ruth
Is that natural language versus AI? Calling in and giving specifics and having it understand? Or is it different?
Sarah
Let’s talk a little bit about that. AI is actually a component that has nothing to do with phones or anything like that. You can use AI across businesses in many different ways.
AI is just a set of patterns and rules that can be applied to a piece of data. That data could be an image, it could be a sound bite, it could be a set of words that come in from a chatbot. It could be any of those things and AI is going to apply some rules across that to say, “what is this? What do I do next? What do I do with this information?” That’s where the artificial intelligence piece comes in.
Let’s say you have 1,000 pictures of a dog. Now, if you show me another picture of a dog, I know it’s a dog because I looked at all the features of a dog in the other photos. Even though it has two ears, I know it’s not a cat because of all the other things around it. You can do that without natural language. I could set up something on my computer to look at, assign the proper rules and, using AI technology, analyze emails coming in and say “if you see all these words together, that means this email is about this and you should take this action.” That’s kind of how we use AI in the contact center space. We take in words from a source, then we have the AI technology pick out what’s important to us and assign it into variables that we can use later.
Even when people don’t use the exact same words or phrasing, AI works. It knows the words people might say around a certain concept. One person can say “I want to transfer money from my checking to my savings account,” and another might say “funds” instead of “money.” The AI engine will still process it correctly because it knows all of the words that people might say around that concept.
AI can also pick out certain pieces of information and, depending on how it hears or sees those words, assign things like a “from” account and a “to” account, maybe even an amount. If a customer says “transfer $500 from checking to savings” the AI knows it’s more than “transfer funds,” it knows the amount and both the “to” and “from” accounts.
That’s the AI part. The natural language part is separate from that, although they usually go hand in hand in a contact center. The natural language piece lets me ask open-ended questions rather than a menu-based, finite lists of options. For example, I might have a phone application that asks “what’s your account number,” and then “what would you like to do today?” Then there is a piece of software that is usually doing a transcription in between. So a phone call comes in, that call is answered, the AI asks the caller a question, and they respond. At that point, the speech-to-text software transcribes the response.
Ruth
Like visual voicemail on an iPhone?
Sarah
Exactly. It’s very similar technology and there are a lot of makers of that technology. Google has a speech-to-text engine. IBM Watson has its own speech-to-text engine. There are some third-party options as well. But it’s really listening to voice and transcribing. And all of that happens first, before AI is even invoked. Because AI doesn’t use WAV files. It doesn’t take a snippet of speech. It only takes transcribed text as an input. That’s the natural language part, that speech-to-text transcription, and then that gets run through the AI component to determine what the person wants. What did they just ask for? All these words in context, what do they mean together? And then those two pieces are put together to build a speech-based conversation. Those are the two components of conversational AI, the AI software and then the natural language put together.
Ruth
I feel like I’ve learned so much in just 10 minutes!
Sarah
Great! I think we make the assumption that people know what they’re asking for when they come to us for projects. They’ll say “I want conversational AI” because it’s kind of a buzzword and it’s new technology. We need to make sure the customer understands what they’re asking for and what they’re buying.
“…you don’t have to cover everything you already have. You can just find one use case for it and go from there.”
Ruth
With projects that you’re currently working on, are you seeing any trends within conversational AI?
Sarah
A lot of it is just people getting their foot in the door right now because the technology is still relatively new. I think a lot of people are asking how they can deploy it in their contact center and trying to figure out a use case for it. I highly encourage anyone who is interested in the technology to figure out how to pick a really good use case.
What I’m also seeing with many of our clients is rather than converting a whole system over and moving everything in their customer environment to full natural language at once, they are starting smaller. For instance, maybe picking a component like an address change or something similar that’s a little bit harder to do in your traditional world. Transfer funds is another great one, because they can speak it up front. I know that a lot of companies are starting to use it for things that were never automated before.
Shoe inventory is a good retail example. It would’ve been impossible before. Inventory changes every week, and you’d need to provide a new weekly inventory list and constantly make updates to the system to be able to support the new information. But now, this is a good use case for conversational AI.
Someone can call in and ask “Hey, do you have these Air Jordan retro shoes?” The conversational AI knows the word “shoes.” If you tell the system about a few shoe brands, like Nike and Adidas, and then a customer mentions another shoe brand, the system is likely going to understand that they are talking about a brand of shoe. You didn’t have to tell it a lot. You just give it a little bit of data to start it out and it’s going to be able to capture that information, too.
Retail businesses are using it for when they don’t have finite lists of things and where maybe inventory is changing constantly. Those are great ways to start out. Again, you don’t have to cover everything you already have. You can just find one use case for it and go from there.
Another trend I’m seeing is simply that customers—end users—are expecting it. One of our clients, who does not have any AI today, has noticed customers calling in and trying to interact with their directed system like it’s a natural language system.
Ruth
They are used to talking to Alexa and Siri and expect that now?
Sarah
Right. They are used to a conversational AI experience before they get to your call center and they want to use it when they get there.
Like, they’ll say, “I have a delivery problem.” We can recognize that. But then when we get into it, the AI will ask them “What date was your problem on?” and instead of them giving just the date, they may say “It was yesterday.” Conversational AI would be able to handle that.
Ruth
I think the big takeaway is that, whereas maybe ten years ago people were more comfortable pressing a button, now, thanks to Siri and Alexa, they’re getting used to just talking and expecting to be understood. That’s a huge shift in consumer behavior.
Sarah
That’s so true. Your customers are expecting you to do this. If you aren’t, you’re letting them down in a way. We’ll have customers come to us and say “well, I love the way company X does it.” I hear that all the time.
.png)
Ruth
Is it true that AI starts to learn things after so many interactions? Is it because callers are saying new things, and those are getting transcribed and it’s being stored as additional information that the AI engine will recognize? Is that how it works?
Sarah
Actually, there’s a couple of ways that can work. First, AI learns on its own. For instance, with the shoe retailer, it already has some rules in it so you don’t have to tell it about every brand of shoes. A person can call in and say most any brand of shoe and it’s going to know what category that’s in. If you want to recognize pieces of clothing, you don’t have to tell it about every piece of clothing. You don’t have to say pants and shirts and socks. You just can tell it about a few pieces of clothing.
And then let’s say someone comes in and says “sweater.” Well, you told it about pieces of clothing and it knows that a sweater is a piece of clothing, so you didn’t have to tell it that sweater was one of the words you wanted it to recognize.
It’s all based on patterns. If the pattern is a type of clothing and someone calls in and says “sweater,” well, that’s a type of shirt and the AI knows it’s clothing. Or if somebody calls in and says “joggers” instead of “pants” the system will recognize them as a type of pants. You don’t have to tell it about those things once you build the initial model. Of course, sometimes there are things that it can’t just automatically categorize.
Ruth
What do you do in those cases?
Sarah
Conversational AI components like IBM Watson or Google have an interface that you can go into and see which words it didn’t map to any intent. Let’s pretend that someone comes in and says “kimono” and maybe that’s just not a word that Google had mapped to clothing type. We’re going to be able to see that it didn’t get mapped to anything. Then, a developer goes in there periodically and, with one click, tells the AI that “kimono” is a type of clothing, and it will recognize it from then on.
Tuning the AI piece—adding additional things to it, making sure it’s working—is really pretty easy. The part that’s a lot harder, and that we don’t have as much control over, is the natural language piece. I’ll give you an example from a clothing retailer that we’ve been working with. We recently made some updates for them for Black Friday.
This customer has a loyalty program. But when people would call in and say “loyalty,” the speech-to-text transcription would read “boil tea” 75% of the time. I think a lot of these companies that provide AI are really good at the AI component, but not necessarily the best at the transcription piece.
That’s why we are always looking for the strongest transcription engine, because the more accurate that is, the better the AI will work. We’ve been exposed to a lot of that stuff already through many real-life scenarios and projects. We know some tips and tricks that we can do to the platform. We know to give a little bit of context to the transcription engine, like telling it that if it hears the word “loyalty” or anything close to it, to transcribe it as “loyalty,” not “boil tea.” We’ve been through some of the growing pains with this technology. It’s not perfect. It’s still new technology.
Ruth
And you definitely want to make sure the AI understands as much as possible, so that the caller on the other end doesn’t get frustrated and start yelling “Representative!”
Sarah
Exactly! If you don’t have a good transcription, the AI is never going to work. It’s the garbage in, garbage out concept.
We used to have to build a statistical language model for NLU and collect somewhere in the range of 20,000 utterances from callers to be able to build a model that made sense. With AI products, I collect about 500 calls instead of 20,000. If you think about the amount of time it takes to transcribe 20,000 calls and then put them into categories–that would take months! That’s why launching a traditional natural language project took one or two years before AI. And now, it’s probably more like six months.
Ruth
Wow, that’s a huge difference!
Sarah
And that’s because by incorporating AI you don’t have to build those statistical language models anymore. I don’t need 20,000 samples to be able to know that I’m doing the right thing. The AI already did that for me repeatedly and trained their model to know about more stuff than I could ever know about in my lifetime. Now, I just have to give it some examples.
We recently added some items for another of our retail clients for Black Friday. We had less than three weeks total, start to finish. Now, if I had to add those words to traditional NLU before AI, there’s no way we would have had even a prayer of getting in the changes!
Ruth
Perfect example. And it’s so timely right now with the holiday shopping season in full swing.
Sarah
Retailers get these spikes of calls that they can’t control. They may have a very manageable call volume for three quarters of the year, even more than three quarters of the year, but the last two months of the year… mid-November, all the way through to December and then into the third week in January because of returns, it spikes.
Conversational AI saves live agents so much time and volume in a period where they are slammed with calls. Even peeling off small percentages of numbers during retailers’ holiday season has a huge impact.
Chatbots get about a 50% containment rate. You go to a website, the chatbot pops up, and you can type your order number and click on a link to get your order details and tracking information and all of that. You can find out if you have a loyalty reward to redeem and get the code for it. All of that sort of stuff. About 50% of the time, the call ends up needing a live agent, but about 50% are deflected. It’s in the 30% range for voice calls—a little bit smaller, but still a good number.
Ruth
Absolutely! Before we wrap up, are there any other instances you’d like to provide about how incorporating NLU and Conversational AI solves problems and improves the customer experience?
Sarah
We are helping another customer expedite their payment process without having to go through a huge number of menus. Callers can say up front how much of a payment they want to make, or they can give us other information.
The challenge is that sometimes customers have multiple lines of business. In this instance, they may have two accounts such as a credit card and an installment loan, but they don’t know their account numbers. AI can recognize the customer using the phone number they’re calling from and know the accounts they have. If the customer is asking about their available credit, the AI system knows this is about their revolving credit account because their other accounts don’t have available credit. So, we’re using keywords that the customer says to figure out which account they actually want to talk about, which is super helpful.
Insurance companies are another example where people have multiple accounts. The conversational AI uses words that the customer said when asked what they’re calling about to determine the appropriate account. It doesn’t have to ask them later. And it’s important because it’s something people aren’t necessarily thinking about because they don’t know it has anything to do with the transaction they want.
Ruth
Thank you so much for taking the time with me today to talk about what Conversational AI is and how it is helping all kinds of different industries!
*This interview has been edited for clarity and length.
Sarah is a featured speaker at Avaya Engage.
Watch Sarah on the on-demand webinar Keys to Creating a Great AI Strategy.
Learn more about Conversational AI or speak to us today!
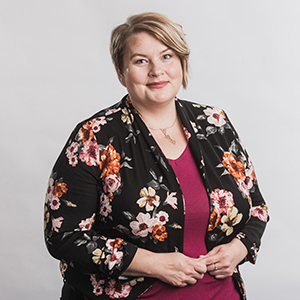
Sarah Reitsma
Director of UI
Waterfield Technologies
As Director of UI at Waterfield Technologies, Sarah is the lead voice strategist in support of Waterfield Tech’s user experience team. She brings over 20 years of speech science and B2C design expertise to the contact center space where she has been instrumental in guiding the transformation of voice and digital experiences for our top clients around the globe.